In today’s digital era, data science has emerged as a cornerstone for business innovation and growth. By harnessing the power of data, businesses can unlock a wealth of insights, driving strategic decisions and operational efficiency. This field, blending expertise in artificial intelligence, algorithms, and various programming languages such as SQL, Python, and R, has become instrumental in transforming raw data into actionable intelligence. The role of a data scientist has thus evolved, becoming a vital asset for organizations looking to thrive in a data-driven landscape.
The synergy of data science and artificial intelligence, coupled with advanced technologies like cloud computing and robust data analysis frameworks, is shaping the future of businesses across sectors. As organizations navigate through vast amounts of data, the integration of these disciplines promises strategic advantages, fostering a culture of innovation and informed decision-making. This article delves into the pivotal role of data science in modern business, exploring its application, benefits, and the emerging trends that are set to redefine industry standards.
The Importance of Data Science and AI in Modern Business
In the contemporary business landscape, the integration of Data Science and Artificial Intelligence (AI) is not merely a technological advancement but a fundamental component that drives strategic decision-making and operational efficiency. These technologies are pivotal in transforming large volumes of data into actionable insights, thereby enhancing business processes and boosting competitiveness.
Transformative Impact on Decision-Making
Data Science and AI significantly influence business decision-making by providing a deep understanding of complex data sets. Through advanced algorithms and machine learning principles, these technologies uncover hidden patterns and correlations that are not apparent through conventional analysis methods. This capability allows businesses to predict consumer behavior, optimize operational processes, and enhance product offerings, ensuring that decisions are not just intuitive but data-driven and analytically sound.
Enhancing Business Operations with AI
AI’s role in modern business extends beyond analysis, deeply embedding itself into daily operations through automation and real-time decision-making. From streamlining supply chains to optimizing resource allocation, AI automates and enhances processes that were traditionally labor-intensive and time-consuming. This not only boosts productivity but also allows human employees to focus on more strategic tasks, adding value to the business.
Strategic Advantages of Data Science and AI Synergy
The synergy between Data Science and AI provides businesses with a strategic edge. Data science offers the tools and techniques to extract valuable insights from big data, while AI amplifies this capability by automating the analysis and interpretation process at speeds and accuracies that humans cannot match. This partnership is crucial in areas like market analysis, customer service, and financial forecasting, where rapid and accurate decision-making can significantly affect business outcomes.
Real-World Applications and Case Studies
In sectors ranging from healthcare to finance, the practical applications of Data Science and AI are vast. For instance, in healthcare, predictive analytics can forecast patient outcomes, enhancing treatment protocols and patient care. In finance, AI-driven algorithms are used for real-time risk assessment, fraud detection, and personalized customer banking experiences, leading to increased customer satisfaction and loyalty.
By harnessing the power of Data Science and AI, businesses not only enhance their operational efficiencies but also foster innovation, tailor customer experiences, and predict future trends. This technological integration is essential for any organization aiming to thrive in the digital age and maintain a competitive advantage in its industry.
Defining Data Science and Its Role in Business Growth
Data science stands as a pivotal force in modern business, harnessing a blend of statistical analysis, machine learning algorithms, and domain expertise to extract actionable insights from vast datasets. This multidisciplinary field not only supports but significantly propels business growth by enabling informed decision-making and strategic planning.
Exploring the Multidisciplinary Approach
The essence of data science lies in its inherent multidisciplinary nature, which integrates various scientific methods and processes to derive meaningful information from data. This approach involves a collaborative effort where data scientists work alongside subject matter experts to ensure the relevance and applicability of data insights across different business domains. By employing advanced computational and statistical techniques, they identify patterns, predict trends, and formulate strategies that are crucial for business advancement.
Data science is not isolated to a single aspect of business operations but spans various departments and functions. From marketing to finance, the insights derived through data science enable businesses to optimize their operations, tailor customer experiences, and innovate product offerings. The role of data scientists is integral in this process, as they utilize their expertise to analyze data, develop predictive models, and implement machine learning solutions that address specific business needs.
The Process of Transforming Data into Insights
Transforming raw data into actionable insights is a structured process that involves several key steps:
- Data Collection and Cleaning: Gathering data from various sources and ensuring it is accurate and free from inconsistencies.
- Data Analysis: Using statistical tools and algorithms to explore and examine the data, uncovering underlying patterns and relationships.
- Insight Generation: Interpreting the analyzed data to produce findings that are directly applicable to business decision-making.
- Implementation: Applying the insights to real-world business scenarios to drive growth and improve efficiency.
This process is cyclical and iterative, allowing businesses to continuously refine their strategies based on new data and evolving market conditions. By effectively leveraging data science, companies can not only react to current trends but also anticipate future challenges and opportunities, positioning themselves favorably in a competitive landscape.
In conclusion, data science is a dynamic and essential element in the toolkit of modern businesses, driving growth and innovation through a deep understanding of data and its implications. By embracing a multidisciplinary approach and rigorously applying data to strategic decision-making, businesses can achieve sustained success and adaptability in the ever-changing business environment.
Understanding Artificial Intelligence and Its Business Applications
Artificial Intelligence (AI) and its subset, Machine Learning (ML), have transcended the realms of science fiction to become key drivers of business innovation. Today, the focus has shifted from fantastical AI characters to practical applications that significantly enhance business operations. AI and ML are particularly adept at tasks like prediction, classification, and generating creative content. They leverage vast data sets, outperforming human capabilities in specific areas such as demand forecasting, customer behavior classification, and product recommendations. This capability not only optimizes inventory and marketing strategies but also enriches customer engagement experiences.
Differentiating between AI and Machine Learning
While AI and ML are often used interchangeably, it’s important to understand their distinct roles within the technological landscape. AI is the broader concept that encompasses the ability of machines to perform tasks that typically require human intelligence. This includes everything from understanding natural language to recognizing patterns in data. ML, on the other hand, is a subset of AI focused on the idea that systems can learn from data, identify patterns, and make decisions with minimal human intervention. Deep learning, an advanced form of ML, utilizes large neural networks to analyze data and learn complex patterns, enabling machines to make predictions independently of human input.
The integration of AI and ML into business systems and strategic plans allows leaders to act on data-driven insights more swiftly and efficiently. This is particularly evident in industries such as manufacturing, where AI automates business processes, and banking, where it secures customer data and enhances service efficiency.
Real-world Applications of AI in Business
AI and ML have found applications across various business functions, streamlining operations and enhancing customer experiences. Here are some notable examples:
- Demand Forecasting: AI systems predict product demand, enabling businesses to manage inventory more efficiently.
- Classification Analysis: AI helps in identifying potential customer churn and segmenting customers for targeted marketing strategies.
- Recommender Systems: These systems improve customer experience by suggesting products that consumers are likely to enjoy.
- Semantic Search: AI enhances customer service by enabling product searches through natural language queries.
- Generative AI: This technology boosts productivity across various business functions, including merchandising, marketing, and customer support.
In light of the COVID-19 pandemic, AI has also played a crucial role in adapting to new consumer behaviors. Customers increasingly seek digital, no-touch interactions with businesses, prompting a shift towards AI-powered touchless interfaces like voice interfaces, facial recognition, and apps. The deployment of AI in businesses is not just about survival in a fast-paced market but thriving in an AI-driven world.
Furthermore, AI’s influence extends to everyday devices and services, from smart assistants like Siri and Alexa to AI-powered platforms like iRobot’s Genius Home Intelligence. These examples underscore AI’s pervasive role in modern life, enhancing efficiency and convenience.
In conclusion, understanding the distinction between AI and ML and recognizing their practical applications in business is crucial for organizations aiming to leverage technology for growth and innovation. AI and ML are not just technological advancements; they are transformative forces reshaping the business landscape, driving efficiency, and fostering a new era of intelligent operations and customer interactions.
Synergizing Data Science and AI for Strategic Advantage
The strategic synergy of data science and artificial intelligence (AI) offers a transformative potential for businesses, enabling them to harness vast amounts of data for enhanced decision-making and streamlined operations. This section explores how integrating these technologies can provide a strategic advantage by enhancing decision-making processes and reducing operational costs.
Enhancing Decision-Making Processes
Data science and AI significantly elevate the decision-making capabilities within an organization by automating the analysis of large datasets and providing insights that are not immediately apparent. For instance, machine learning algorithms can predict consumer behavior, optimize business operations, and enhance financial forecasting. This allows companies to make informed decisions quickly, staying ahead in competitive markets.
- Predictive Analytics: AI-driven predictive analytics empower organizations to foresee market trends and consumer needs, allowing them to align their products and services strategically.
- Real-Time Insights: With AI, businesses can process and analyze data as it is collected, enabling real-time decision-making that can dramatically improve responsiveness to market dynamics.
- Risk Assessment: Advanced AI algorithms help in identifying potential risks by analyzing patterns that could lead to future problems, thus allowing businesses to mitigate risks proactively.
Streamlining Operations and Reducing Costs
AI not only enhances decision-making but also plays a pivotal role in optimizing business operations, which can lead to significant cost reductions.
- Automating Routine Tasks: AI technologies like Robotic Process Automation (RPA) take over repetitive, time-consuming tasks such as data entry and invoice processing, freeing up human resources for more strategic activities.
- Operational Efficiency: AI-driven systems improve operational efficiency by optimizing logistics, supply chain management, and inventory control, reducing waste and downtime.
- Predictive Maintenance: Utilizing AI to predict equipment failures before they occur prevents costly downtime and extends the lifespan of machinery.
By integrating AI into their workflows, businesses not only streamline operations but also enhance their strategic decision-making capabilities. This dual advantage is crucial in leveraging the full potential of both data science and AI for achieving sustainable growth and maintaining a competitive edge in today’s data-driven economy.
Key Benefits of Data Science and AI Integration
Predictive Analytics for Forecasting
Predictive analytics harnesses historical data and statistical models to forecast future trends, enhancing strategic decision-making across various business domains. This capability allows businesses to anticipate market demands, consumer behavior, and potential operational challenges effectively. Here are some specific ways in which predictive analytics proves beneficial:
- Market Research: By analyzing past market trends and consumer behavior, businesses can predict future market conditions and consumer preferences, tailoring their products and marketing strategies accordingly.
- Product Development: Insights from predictive analytics enable companies to innovate and develop products that meet anticipated customer needs, staying ahead of market trends.
- Enhanced Marketing: Utilizing predictive analytics in marketing efforts helps in identifying the most effective strategies and optimizing marketing campaigns for better reach and engagement.
These applications of predictive analytics not only streamline operations but also provide a competitive edge by allowing businesses to act proactively rather than reactively.
Automating Repetitive Tasks and Customer Service
The integration of AI in automating routine business processes and enhancing customer service operations offers significant efficiency and satisfaction improvements. AI-driven tools such as chatbots and automated ticketing systems streamline customer interactions and support workflows, providing several key advantages:
- Efficiency in Customer Service: AI-powered chatbots handle routine inquiries and support tickets, reducing the workload on human agents and allowing them to focus on more complex issues. This leads to faster resolution times and increased customer satisfaction.
- Cost Reduction: Automating repetitive tasks cuts down on labor costs and operational expenses. AI systems can manage these tasks continuously without the need for breaks or downtime, optimizing resource allocation.
- Scalability: As businesses grow, AI systems can easily scale to handle an increasing volume of tasks and customer interactions without the need for proportional increases in human resources.
These benefits underscore the transformative impact of AI and data science on business operations, driving efficiency, reducing costs, and improving overall customer experiences. By leveraging these technologies, businesses can achieve streamlined operations and enhanced decision-making capabilities.
Emerging Trends in Data Science and AI
The Rise of Automated Machine Learning (AutoML)
Automated Machine Learning (AutoML) is transforming the landscape of data science by making advanced machine learning techniques more accessible and efficient. AutoML automates the process of applying machine learning to real-world problems, simplifying the tasks of model selection, feature engineering, and hyperparameter tuning. This technology enables businesses of all sizes to harness the power of machine learning without the need for extensive expertise.
- Increased Accessibility: AutoML tools are designed to be user-friendly, allowing non-experts to implement machine learning models effectively. This democratization of technology empowers more employees to engage in data-driven decision-making.
- Enhanced Productivity: By automating routine data science tasks, AutoML frees up skilled professionals to focus on more strategic and innovative activities.
- Cost Efficiency: Reducing the reliance on human intervention for building and tuning models can lead to significant cost savings, particularly in reducing the time to deploy machine learning solutions.
Quantum Computing’s Impact on Data Processing
Quantum computing is poised to revolutionize data processing capabilities in data science and artificial intelligence. With its ability to perform calculations at unprecedented speeds, quantum computing offers a significant advantage in handling large volumes of data and complex computations.
- Speed and Efficiency: Quantum computers can process information at speeds unattainable by classical computers, dramatically reducing the time required for data analysis and insight generation.
- Advanced Problem Solving: Quantum algorithms are uniquely suited to solving complex optimization problems and simulations, providing more accurate and nuanced insights for decision-making.
- Enhancement of AI Models: The integration of quantum computing with AI can lead to the development of more sophisticated models, capable of faster learning and more precise predictions.
These emerging trends in data science and AI, represented by AutoML and quantum computing, are not only enhancing current practices but are also setting the stage for future innovations that could transform entire industries. By leveraging these technologies, businesses can gain a competitive edge through more effective data utilization and decision-making processes.
Building a Data-Driven Culture in Your Organization
Building a data-driven culture within your organization is a transformative journey that requires commitment, strategic planning, and continuous learning. It’s about empowering every team member, regardless of their role or background, with the ability to explore, understand, and communicate with data meaningfully. This journey not only enhances decision-making and innovation but also positions your organization for sustainable growth and competitive advantage.
Fostering Data Literacy Among Employees
- Develop a Comprehensive Skill Set:
- Technical Skills: Equip your team with the ability to work with data effectively. This includes data analysis, data visualization, and data storytelling. Employees should understand data sources and quality, interpret visualizations and analytics, and communicate data insights. Familiarity with tools and platforms like Excel, Power BI, Pyramid Analytics, and SQL is essential.
- Non-technical Skills: Encourage critical and creative thinking with data. This involves problem-solving, research, and collaboration. Your team should define data goals and questions, evaluate data sources, and apply data insights to your business context. Working with others who have different data skills and learning from their feedback is crucial.
- Incorporate Organizational and Individual Practices:
- Organizational Practices: Create a data-literate environment through data governance, data ethics, and a shared vision for data. Establish clear policies on data collection, storage, and use. Align your data strategy with your business goals and incentivize data-driven decision-making.
- Individual Practices: Encourage self-assessment, self-learning, and self-reflection. Team members should be aware of their data skills and seek opportunities to enhance them. Curiosity and open-mindedness about data, along with challenging assumptions, are key to personal growth in data literacy.
Encouraging Innovation and Experimentation
- Cultivate a Culture of Experimentation:
- Embrace an environment where curiosity thrives, risks are encouraged, and failures are seen as stepping stones to success. This approach fosters creative problem-solving, product innovation, and continuous improvement.
- Apply the experimental method systematically through observation, hypothesis formulation, experimentation, data collection, analysis, and conclusion.
- Implement Data-Driven Decision Making:
- Encourage risk-taking, learning from failures, and embracing iterative processes. Create an environment where employees feel empowered to explore new ideas and challenge existing norms.
- Make data-driven decision-making a fundamental practice by gathering and analyzing data. Experiments provide insights and empirical evidence that inform strategic choices, enhancing decision accuracy and effectiveness.
- Provide Necessary Tools and Infrastructure:
- Invest in technology and resources that support experimentation, such as prototyping tools, data analytics platforms, and collaboration software. This infrastructure is crucial for facilitating a culture of experimentation.
- Foster Open and Transparent Communication:
- Establish communication frameworks that promote open dialogue across all levels of the organization. Ideas and feedback should flow freely, encouraging a collaborative and inclusive data culture.
By focusing on fostering data literacy among employees and encouraging innovation and experimentation, organizations can unlock the full potential of their teams. This strategic approach not only drives informed decision-making but also cultivates a dynamic environment where data literacy, creativity, and strategic thinking thrive.
Selecting the Right Tools and Technologies for Your Business
In the fast-evolving landscape of data science and artificial intelligence, selecting the right tools and technologies is not just about leveraging the latest advancements but about finding solutions that align perfectly with your business needs and objectives. This involves a thorough understanding of your project’s goals, the nature of the data you’re dealing with, and the specific challenges you anticipate. Here, we delve into the critical aspects of assessing requirements and compatibility, alongside the undeniable importance of scalability and ease of use, to guide businesses in making informed decisions.
Assessing Requirements and Compatibility
- Understanding Project Goals: The first step in selecting the right tools involves a clear identification of what your data science project aims to achieve. Whether it’s gaining insights, predicting trends, or solving specific problems, the tools you choose should directly support these objectives.
- Analyzing Data Characteristics: Knowing the volume, variety, and complexity of your data is essential. Tools that excel in handling large volumes of structured data might differ from those better suited for unstructured data sets.
- Anticipating Challenges: Consider potential technical limitations, budget constraints, or time frames that could impact your project. Tools that offer solutions to these anticipated challenges should be prioritized.
- Skill Level of Users: The technical proficiency of the individuals using these tools plays a crucial role. Tools need to be accessible to users at different skill levels, from beginners to advanced practitioners.
- Integration with Existing Systems: It’s vital to choose tools that seamlessly integrate with your current data science software or systems. This ensures a smooth workflow and prevents compatibility issues.
- Privacy and Security: For projects dealing with sensitive data, the chosen tools must adhere to strict privacy and security standards to protect data integrity and comply with regulatory requirements.
The Importance of Scalability and Ease of Use
- Scalability: As businesses grow, so do their data science needs. Tools must be able to scale in terms of data volume and complexity. This means they should allow for the addition of new features or integration with other systems without causing disruptions. Scalability ensures that as your business evolves, your data science capabilities can grow accordingly, preventing the need for frequent tool replacements.
- Ease of Use: Tools should be intuitive and user-friendly, minimizing the learning curve for new users. This is particularly important in fast-paced business environments where time is of the essence. Tools with interactive programming features or drag-and-drop functionality can significantly enhance productivity and allow users to focus more on analysis and less on figuring out how to use the software.
- Reliability and Documentation: The results produced by your data science tools must be reliable enough to inform business decisions. Additionally, quality documentation is crucial as it provides clear instructions on using the software and detailed information on its features and capabilities. This enables users to understand and effectively utilize the tools in their projects fully.
Selecting the right tools and technologies for your business in the realms of data science and AI is a strategic decision that can significantly impact your organization’s ability to derive actionable insights and maintain a competitive edge. By carefully assessing requirements and compatibility, and prioritizing scalability and ease of use, businesses can ensure they invest in solutions that not only meet their current needs but are also poised to support future growth and innovation.
Strategies for Successful Data Science and AI Integration
Aligning with Business Objectives
Successfully integrating data science and AI into business operations begins with a clear alignment between data strategies and business objectives. This alignment ensures that every data initiative directly supports the overarching goals of the organization, enhancing decision-making and operational efficiency.
- Understanding Business Goals: Start by identifying the core objectives of the business, such as increasing revenue, improving customer satisfaction, or optimizing operational processes. This step is crucial for defining the scope and direction of data science applications.
- Identifying Relevant Data: Determine which types of data are most relevant to the identified business goals. This includes both internal data, like sales figures and customer interactions, and external data, such as market trends and demographic information.
- Evaluating Infrastructure: Assess the current data management infrastructure to ensure it can support the proposed data science and AI initiatives. This may involve upgrading existing systems or investing in new technologies.
- Setting KPIs and Metrics: Establish clear key performance indicators (KPIs) that will help measure the success of data science projects. These metrics should be directly linked to business outcomes to assess the impact of data-driven strategies accurately.
- Fostering Data-Driven Culture: Encourage a culture that embraces data-driven decision-making. This involves training employees to use data effectively and making data accessible across the organization to foster an environment of informed decision-making.
Overcoming Implementation Challenges
Implementing data science and AI technologies involves navigating several challenges that can hinder their effective integration. Addressing these challenges head-on is crucial for leveraging the full potential of data-driven technologies.
- Ensuring Data Quality: High-quality data is the foundation of effective AI applications. Invest in robust data management practices such as data cleaning, normalization, and integration to ensure the data used is accurate and comprehensive.
- Bridging Talent Gaps: The demand for skilled data scientists often outstrips supply. Develop strategies for talent acquisition, such as partnering with universities or offering internal upskilling programs, to build a competent data science team.
- Integrating with Existing Systems: Seamless integration of AI tools with existing business systems is essential. Choose AI solutions that are compatible with current infrastructure and plan for gradual integration to minimize disruption.
- Addressing Ethical and Legal Issues: Navigate the ethical and legal implications of AI by establishing clear policies on data usage, privacy, and security. Regular audits and compliance checks should be conducted to adhere to regulatory standards.
- Scaling AI Solutions: Ensure that AI systems are scalable and can grow with the business. This involves investing in flexible and adaptable technologies that can accommodate increasing data volumes and complexity.
By strategically aligning data science initiatives with business objectives and effectively managing the implementation challenges, organizations can enhance their operational capabilities and drive significant business growth through informed data-driven decisions.
Conclusion
Throughout this exploration, it has become evident that data science and artificial intelligence (AI) are more than mere technological advancements; they are pivotal forces driving the future of business. By weaving together the threads of predictive analytics, automated machine learning, and strategic integration, businesses stand on the cusp of a transformation that promises increased operational efficiency decision-making prowess, and a competitive edge in the ever-evolving market. The dynamic interplay between data science and AI, bolstered by a culture of innovation and a commitment to data-driven decision-making, heralds a new era of business strategy and development.
As organizations navigate the complexities of data science and AI integration, the journey is underscored by the necessity of continuous learning, adaptation, and alignment with core business values and objectives. The potential of these technologies to revolutionize business practices, propel growth, and foster sustainable competitive advantage cannot be overstated. By harnessing the power of data science and AI, businesses are not just preparing for the future; they are actively shaping it, setting a foundation for innovation, resilience, and unparalleled success in the digital age.
FAQs
-
How does data science influence the future of businesses?
Data science plays a crucial role in enhancing business operations by providing deep insights into customer preferences, behaviors, and needs. By utilizing this valuable information, companies can tailor their products, services, and marketing strategies to align more closely with customer expectations. This personalization fosters greater customer satisfaction and loyalty.
-
In what ways will data science evolve to shape the future?
Data science is set to deeply integrate with advancements in artificial intelligence (AI) and machine learning (ML). This integration will enhance the capabilities of data science, enabling more sophisticated analyses and predictive capabilities. Such advancements will allow for the automation of complex processes and facilitate more precise predictions on a larger scale.
-
What contributions does data science make to business operations?
Incorporating data science into business operations significantly enhances productivity, decision-making, and product development. It is a powerful tool to reduce or eliminate risks associated with fraud and errors, boost operational efficiency, and improve customer service.
-
What role does data science play in business decision-making?
Data science is instrumental in predicting trends and future outcomes and optimizing decision-making processes. By analyzing historical data, data science helps businesses identify patterns and trends, enabling them to make well-informed predictions about market changes, customer demands, and shifts within the industry.
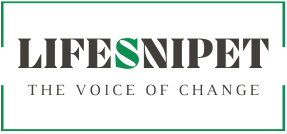
Welcome to LifeSnipet! At LifeSnipet, we’re your ultimate source for the latest health updates. Specializing in health and fitness-related diseases, we delve deep into Ayurvedic techniques, providing you with a comprehensive understanding of well-being. Explore our real-time updates, detailed articles, and ancient Ayurvedic wisdom for a holistic approach to health. Embark on a journey to a healthier, vibrant life with LifeSnipet – where your well-being is our priority!